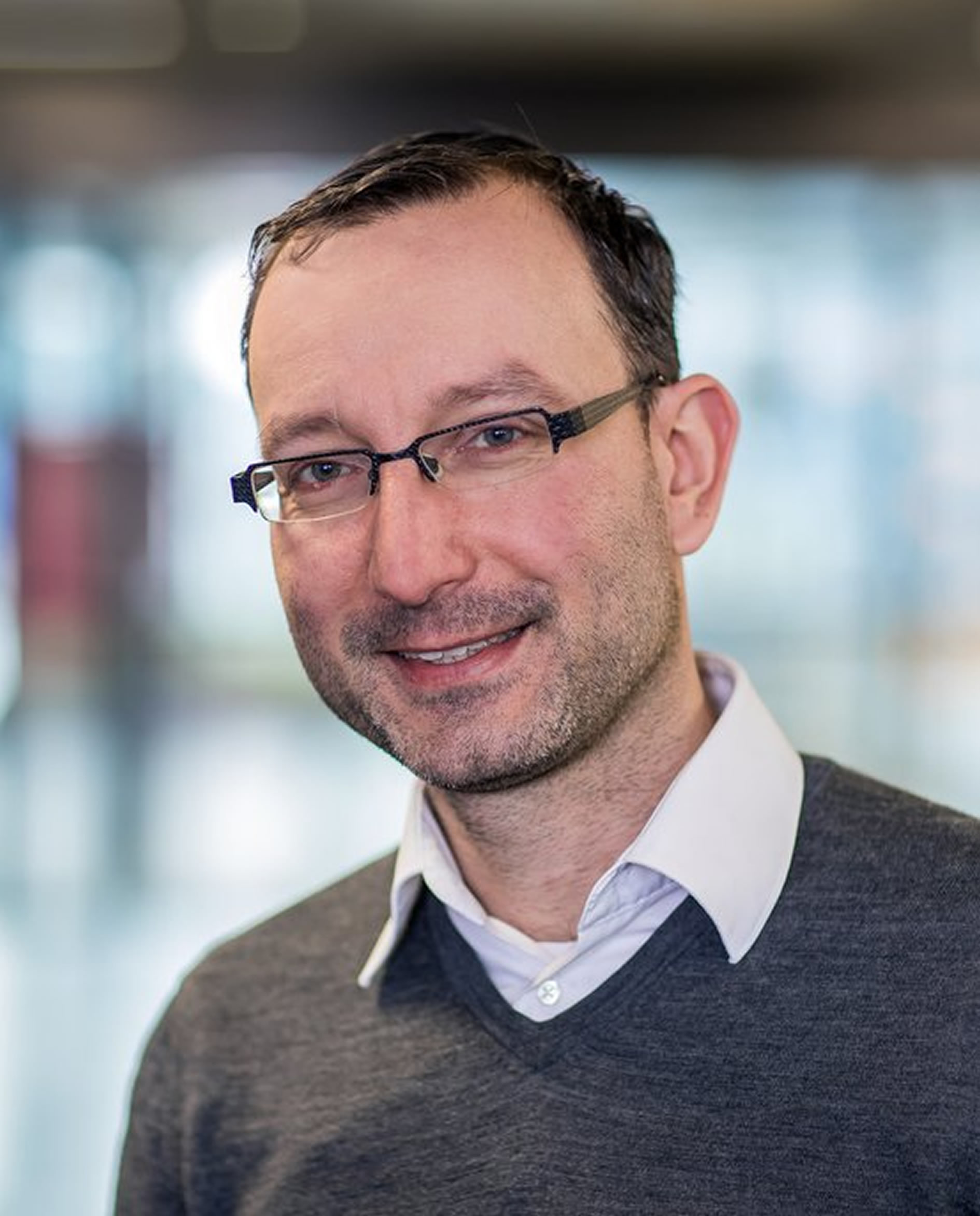
The Interplay Between High-Level Problems and the Process Instances that Give Rise to Them
Bakullari, B., Thoor, J. V., Fahland, D., & Aalst, W. M. P. v. d. (2023). The Interplay Between High-Level Problems and the Process Instances that Give Rise to Them. In BPM (Forum) (pp. 145-162) https://doi.org/10.1007/978-3-031-41623-1_9